In the age of artificial intelligence (AI), the ability to understand and trust AI models is essential for their widespread adoption and acceptance. Enter explainable AI models – a groundbreaking approach that enhances transparency and trustworthiness by providing insights into how AI algorithms make decisions. In this blog, we'll explore the significance of explainable AI models, their applications across various industries, and the role they play in fostering trust and accountability in AI systems.
Understanding Explainable AI: Shedding Light on Black Box Models
Explainable AI refers to the ability of AI systems to provide explanations for their decisions and predictions in a clear and interpretable manner. Traditional AI models, often referred to as "black box" models, operate in a manner that is opaque and difficult to interpret. This lack of transparency raises concerns about bias, fairness, and accountability, particularly in high-stakes applications such as healthcare, finance, and criminal justice. Explainable AI models address these concerns by providing insights into the underlying factors and reasoning behind AI-driven decisions, enabling users to understand, interpret, and trust the outputs generated by AI systems.
Enhancing Transparency and Accountability in AI Systems
Explainable AI models enhance transparency and accountability in AI systems by providing visibility into the decision-making process. By revealing the features, patterns, and correlations that drive AI-driven decisions, explainable AI models enable users to assess the reliability and fairness of AI outputs. This transparency not only builds trust in AI systems but also allows users to identify and address biases, errors, and ethical concerns that may arise. In applications such as loan approvals, medical diagnosis, and autonomous vehicles, explainable AI models empower users to make informed decisions and mitigate potential risks, ultimately leading to more responsible and ethical AI implementations.
Applications of Explainable AI Across Industries
Explainable AI models have wide-ranging applications across industries, where transparency and trust are paramount. In healthcare, explainable AI plays a crucial role in assisting clinicians in diagnosing diseases, recommending treatments, and interpreting medical imaging data. Generative AI in healthcare, coupled with explainable AI models, enables clinicians to understand how AI algorithms arrive at diagnostic decisions, facilitating collaboration and ensuring patient safety. Similarly, in finance, explainable AI models help financial institutions assess creditworthiness, detect fraud, and make investment decisions with confidence. By providing explanations for credit scores, investment recommendations, and risk assessments, explainable AI models enhance transparency and accountability in financial decision-making processes.
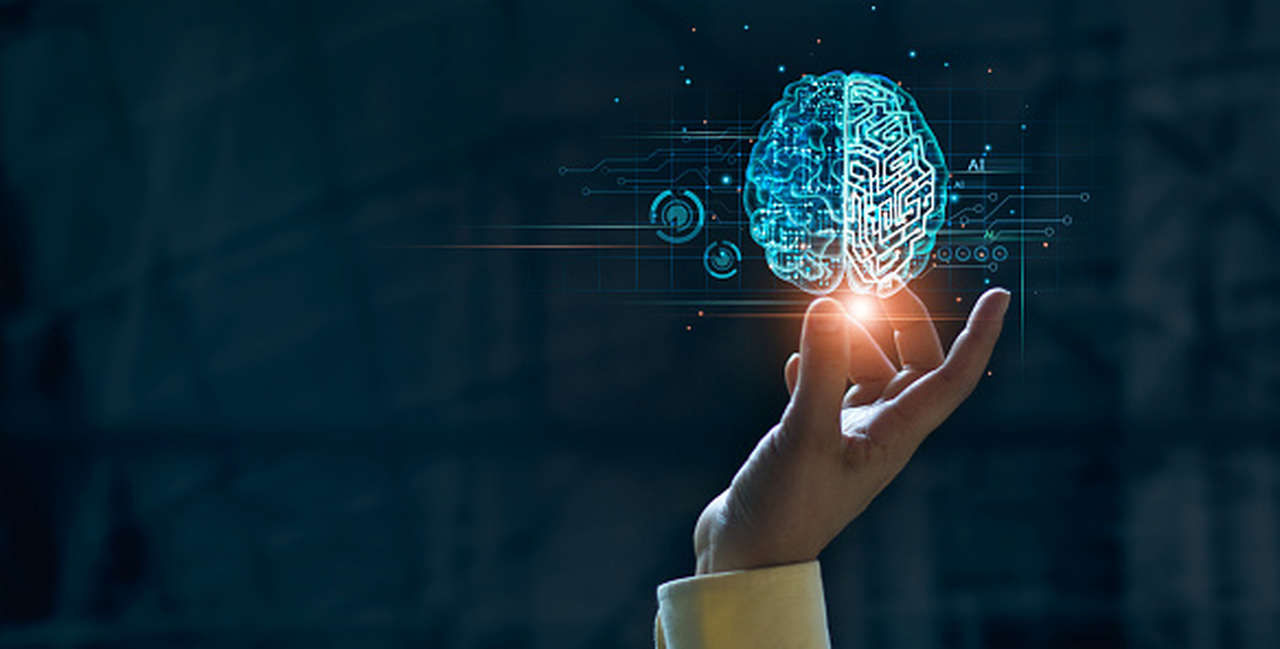
Leveraging Explainable AI in Personalized Learning
In the realm of education, explainable AI models are transforming personalized learning experiences by providing insights into student performance, learning preferences, and educational outcomes. Personalized learning platforms leverage explainable AI algorithms to analyze student data, identify areas for improvement, and recommend tailored learning activities and resources. By explaining how recommendations are generated and why specific learning strategies are suggested, explainable AI models empower educators and learners to make informed decisions about their educational journey. Whether it's adapting lesson plans, providing feedback, or selecting appropriate learning materials, explainable AI enhances the effectiveness and relevance of personalized learning experiences.
Driving Innovation in AI Chatbot Development Services
Explainable AI models are also driving innovation in AI chatbot development services, where transparency and trust are essential for effective communication and interaction. Chatbots equipped with explainable AI capabilities provide users with insights into how responses are generated and decisions are made, enhancing the user experience and fostering trust in AI-powered interactions. Whether it's answering customer inquiries, providing recommendations, or assisting with troubleshooting, explainable AI chatbots enable users to understand and trust the decisions made by AI algorithms. This transparency not only improves user satisfaction but also enables organizations to identify and address potential issues or biases in chatbot interactions.
Addressing Bias and Fairness in AI Systems
One of the key benefits of explainable AI models is their ability to address bias and promote fairness in AI systems. Bias in AI algorithms can lead to unfair or discriminatory outcomes, particularly in applications such as hiring, lending, and criminal justice. Explainable AI models enable users to identify and mitigate bias by providing visibility into the factors that influence AI-driven decisions. By examining the underlying data and features used by AI algorithms, users can assess whether decision-making processes are fair and equitable. This transparency facilitates the detection and correction of biases, ensuring that AI systems produce outcomes that are unbiased and reflective of diverse perspectives.
Empowering Users to Make Informed Decisions
Explainable AI models empower users to make informed decisions by providing them with insights into the rationale behind AI-driven recommendations and predictions. Whether it's selecting a medical treatment, making a financial investment, or choosing an educational pathway, users can better understand the risks and benefits associated with AI-driven decisions. By transparently communicating the factors that influence decision-making, explainable AI models enable users to weigh the evidence, evaluate alternatives, and make decisions that align with their goals and values. This empowerment fosters autonomy and agency, enabling individuals to take control of their decisions and outcomes in AI-driven environments.

Facilitating Collaboration and Knowledge Sharing
Explainable AI models facilitate collaboration and knowledge sharing among stakeholders, enabling interdisciplinary teams to work together more effectively. In fields such as healthcare and finance, where decisions impact multiple stakeholders, explainable AI models provide a common language for communication and understanding. Clinicians, researchers, and policymakers can collaborate more seamlessly by sharing insights into how AI algorithms arrive at decisions, fostering trust and alignment around shared goals. Similarly, in education, explainable AI models enable educators to collaborate with students and parents, providing transparent insights into student progress and learning outcomes. This collaborative approach enhances communication, fosters consensus-building, and drives collective action towards common objectives.
Driving Innovation and Ethical AI Development
Explainable AI models are driving innovation and promoting ethical AI development by encouraging transparency, accountability, and responsible use of AI technologies. By shedding light on the inner workings of AI algorithms, explainable AI models facilitate ethical decision-making and encourage best practices in AI development and deployment. Researchers and developers can use explainable AI techniques to identify and address potential risks, biases, and unintended consequences early in the development lifecycle. This proactive approach minimizes the likelihood of harmful outcomes and fosters a culture of ethical AI innovation. As the demand for ethical AI continues to grow, explainable AI models will play a crucial role in shaping the future of AI technology and ensuring that AI systems are developed and used in a manner that is ethical, transparent, and beneficial for society.
Conclusion: Embracing Transparency and Trust in AI
In conclusion, explainable AI models are revolutionizing the way we understand, interpret, and trust AI-driven decisions. By providing insights into the decision-making process, explainable AI enhances transparency, fosters accountability, and builds trust in AI systems across various industries. From healthcare and finance to education and customer service, the applications of explainable AI are vast and transformative. As we continue to harness the power of AI to drive innovation and solve complex problems, it is essential to prioritize transparency and trustworthiness in AI implementations. By embracing explainable AI models, we can ensure that AI systems are not only intelligent but also ethical, responsible, and trustworthy, ultimately leading to more inclusive and beneficial outcomes for society as a whole.